Mingyi Zhou, Ph.D.
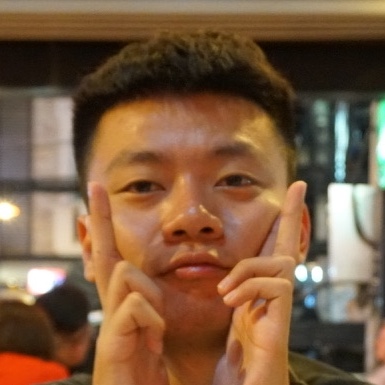
I obtained my Ph.D. from Monash University in Dec 2024. From 2021 to 2024, I have the privilege of being mentored by Prof. Li Li, Prof. John Grundy, Prof. Chunyang Chen, and Dr. Xiao Chen (in a sequence of the length of supervision) at Monash HumaniSE Lab (leading by Prof. John Grundy) and SMAT Lab (leading by Prof. Li Li). Prior to joining Monash University, I worked with Prof. Yipeng Liu and Prof. Ce Zhu in UESTC from 2017 to 2021.
My current research interests include SE4AI, AI4SE, mobile software engineering, AI security, program analysis and computer vision, especially for the on-device AI security and efficiency. If you are interested in my research, please feel free to contact me.
news
Mar 26, 2025 | Two papers has been accepted by FSE’25 Industry Track! Congratulations to Farong, Zhihao, and the team. ![]() ![]() |
---|---|
Jan 25, 2025 | My PhD thesis “Towards Improving the Reliability of Deployed Deep Learning Software” has been released! ![]() ![]() |
Jan 10, 2025 | Our paper of the first static analysis framework “ArkAnalyzer” for OpenHarmony has been accepted by ICSE’25-SEIP and open sourced. Congratulations to Haonan and the team, welcome to use and cite it! ![]() ![]() |
Nov 06, 2024 | Our paper “LLM for Mobile An Initial Roadmap” has been accepted by ACM TOSEM! Congratulations to Daihang and the team. ![]() ![]() |
Aug 07, 2024 | One paper about dynamic model obfuscation has been accepted by ASE’24! ![]() ![]() |
selected publications
- [FSE’25 Insustry] HapTest: The Dynamic Analysis Framework for OpenHarmony2025
- [FSE’25 Insustry] HapRepair: Learn to Repair OpenHarmony Apps2025